Immunology and Machine Learning Online Symposium
The world’s leading researchers joined Immunai’s second symposium dedicated to applications of Machine Learning (ML) in biology. The line-up of talks demonstrated innovative research taking place at the intersection of immunology and machine learning. ML is becoming an essential tool in every aspect of immunology. Our speakers shared their work on how they leveraged ML in their research, from building better quality datasets to conceptualizing dynamic changes in cell states to in silico experimentation at scale. The symposium was moderated by Immunai’s Chief Science Officer, Jacques Banchereau, and Senior Director of Single-Cell Immunology, Adeeb Rahman.
High-quality datasets yield better ML predictions
Machine Learning starts with data. As Andrew Ng says, ‘Data is Food for AI’. Without good quality data it is nearly impossible to get meaningful results from machine learning models. That is why having large and good quality datasets is an essential part of any ML effort.
Rahul Satija, one of the speakers, dove into why building a large dataset of immune cells can help with exploring the functional role of DNA elements in single cells. Rahul serves as a Core Faculty Member at the New York Genome Center (NYGC) as well as an Associate Professor at the Center for Genomics and Systems Biology at New York University (NYU).
He presented a new single-cell technique called scCUT&Tag-pro, which he and his team, in collaboration with the NYGC Technology Innovation Lab, have used to build a multi-modal atlas of immune cells.
The CUT&Tag technique, on which Rahul’s single-cell method is based, can detect open-chromatin regions (OCRs) that are bound by specific chromatin factors. OCRs are indicative of actively transcribed DNA regions and are thus proxies of chromatin transcriptional activity. scCUT&Tag-pro provides simultaneous measurements of CUT&Tag profiles of specific chromatin factors involved in gene active transcription or repression, as well as cell surface protein levels in single cells. The protein level measurements have allowed them to use data mining techniques to stitch together all the datasets they have collected – gene expression, surface protein binding, chromatin accessibility, and scCUT&Tag-profiles of 6 different chromatin factors – into an atlas of what Rahul calls mega-omic single cell profiles (Figure 1).
His team models the functional state across the genome using a model they call single-cell chromHMM, an extension of chromHMM, a Hidden Markov Model tool for learning chromatin states.
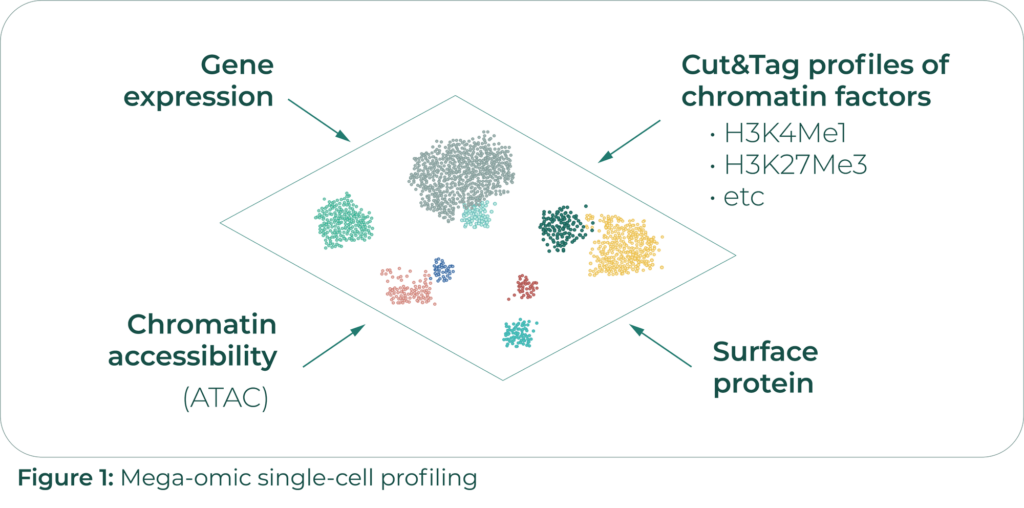
Additionally, they have validated this work by comparing their multi-modal profiles to profiles from Chromatin Immunoprecipitation Sequencing(ChIP-Seq), which is the standard way to measure direct protein interactions with DNA. Their atlas also correctly identifies the maturation cycles of T cells and allows them to answer questions like, “How many regions of DNA are changing in function over the course of maturation?”
Rahul’s research shows how we can dramatically improve the quality of existing datasets by combining their modalities using data science.
Simplicity is a friend of machine learning
Very often, in order to receive great results in machine learning applications, it is not necessary to use complex and sophisticated algorithms. In many cases, they make things needlessly complicated and difficult to interpret. Samantha Riesenfeld,Assistant Professor of Molecular Engineering and Genetic Medicine from The University of Chicago, provides a fantastic example of how machine learning algorithms like topic modeling can help us discover new biological paradigms.
In her research, Samanthamakes a strong case for considering cellular transcriptional profiles as continuous and dynamic states of a cell. This is in opposition to the conventional view of cells as exhibiting discrete phenotypes.
Samantha and her team have been studying innate lymphoid cells. These cells, which are primarily found in barrier tissues like our skin, are traditionally thought to come in specialties – ILC1 cells fight viruses, ILC2 cells fight parasites, and ILC3 cells fight bacteria and fungi.
Psoriasis can be often characterized by inflammation due to a dysregulated immune response. The inflammation corresponds to high ILC3 density in the inflamed regions. However, the mechanisms through which the ILC3 cells arrive in the inflamed regions is not known.
Further analysis and experimental validations suggest that there is a rich spectrum of ILC phenotypes and that some of these ILC cells transition into an ILC3 state, which is what causes the inflamed response.
Samantha and her team have modeled this behavior using topic modeling. Topic modelling is commonly used to classify textual data in terms of its semantic content. It decomposes textual documents into convex combinations of possible topics that they could pertain to. Specifically, Samantha and her team are using a machine learning technique known as Latent Dirichlet Allocation (LDA). It is the most commonly used approach in extracting ‘topics’ (hidden semantics) in a given corpus of data.
They chose this approach because it is simple and immediately interpretable (Figure 2). They use 17 topics, with each topic representing a gene program (a probability distribution over expressed genes). Each cell is modeled as a probability distribution over these topics.

They have run experiments in which they injected the IL-23 inflammatory signaling cytokine into the ear skin of mice every day for 4 days. This caused inflammation analogous to a true psoriasis lesion. They collected tissue samples from biological replicates, isolated ILCs from these samples, and performed single-cell RNA sequencing on the ILCs.
Instead of working directly with the sparse matrix relating each of the thousands of cells to each of the thousands of genes in question, they modeled each cell by its topic distribution. Their analysis consisted of identifying trajectories describing how cells transformed over time in topic space.
Samantha’s team identified common transitions of cells from an ILC2-like state into an ILC3-like state and from a quiescent state into an ILC3-like state. These common transitions suggest that cells at the site of inflammation are transforming into ILC3 cells rather than the ILC3 cells arriving from somewhere else.
Samantha’s work shows how machine learning can refine and improve our understanding of cell states.
The cutting-edge use of ML for experiments at scale
The opportunity to run in silico experiments using ML, saving millions of dollars and bringing reproducibility to experimentation, is one of the most exciting aspects of applying Machine Learning to biology.
Sara Mostafavi, an Associate Professor at the Paul Allen School of Computer Science and Engineering at the University of Washington (UW), discussed the problem of understanding how a single genome can give rise to numerous cell and tissue types. The immune system provides great context for this research because of the diversity of immune cells and because of how quickly they respond to changes in their environment.
Sara’s work builds off of the ImmGen ATAC Atlas Project, which contains ~500k Open Chromatin Regions (OCRs) recorded for ~80 cell types.
Mostafavi’s lab, in collaboration with the Benoist-Mathis lab at Harvard, has developed a convolutional neural network model, AI-TAC, which infers chromatin accessibility in different cell types solely by using regulatory DNA sequences as inputs (Figure 3).

In addition to validating AI-TAC on the ImmGen dataset, Mostafavi and team have also validated it using an ATAC-Seq dataset containing 400k open chromatin regions across 25 human immune cell types.
Through running experiments on validation, interpretation, and reproducibility, they have also identified stable motifs – groups of regulatory sequences which activate several nodes in the first layer of their neural network and whose predictive power survives even the deletion of any small number of nodes from that layer.
Most of these stable motifs correspond to known transcription factors binding sites, providing additional validation of their model. Surprisingly, Mostafavi’s team has also discovered some stable motifs which do not yet map to known transcription factors. This is an active direction of research for Sara and her group.
We are really excited about Sara’s work because it shows how machine learning can drive in silico experimentation in immunology. It also shows the power of machine learning in connecting sequenceable data (DNA) to gene activity and, thus, phenotype.
This symposium demonstrated how machine learning is pushing the boundaries of our understanding of immunology. Gradually, with further scientific and machine learning advancements, we will get even closer to fully leveraging the immune system to potentially fight serious diseases.